Betting and other speculative markets have been around for many centuries, and for many decades economists have studied the ability of such markets to aggregate information. The main finding of this research is that such markets tend to be relatively “efficient” in the sense that it is hard to find information that has not been incorporated into market prices (Lo, 1997; Hausch, Lo, & Ziemba, 1994). The main possible exceptions seem to be long-term aggregate price movements, and a long-shot bias in high-transaction-cost betting markets. Manyhavesuggestedthat asset marketshave too much long-termaggregateprice variation, such as stock market “bubbles” (Shiller, 2000). Risk and delay most discourage speculators from correcting such pricing errors, and irrational traders can actually gain superior returns (though not utility) from irrationally-large risk-taking (De Long, Shleifer, Summers, & Wald- mann, 1990). Long-term aggregate prices, however, are also where it is hardest to empirically distinguish irrationalityfrom rational informationabout fundamental economic change (Barsky & De Long, 1993), and where selection effects most pollute our data (Jorion & Goetzmann, 2000). Even if speculative markets are distorted by irrational bubbles, it is not clear that any of our other information institutions do better. For example, no other information institution in our society, such as academia or news media, consistently predicted that we were over-investing during the “dotcom” bubble. Yes some individual academics or reporters so predicted, but so did some individual stock investors. Over the last few decades economists have also studied speculative markets in laboratory experiments, where they have more control over trader information and preferences. Such experiments find that speculative markets aggregate information well, even with four traders trading $4 over four mintues, and even when such traders know little about their environment or other traders (Sunder, 1995). For example, traders can aggregateinformation well when they are experienced in their role and abstractly know the payoffs of players in other roles (Forsythe &Lundholm, 1990). If the structure of traders’ information is complex enough relative to the number of assets available to trade, however, information “traps” can occur where individual traders have no direct incentive to reveal their information (Noeth, Camerer, Plott, & Webber, 1999). Such problems are typically, though not always, reduced by allowing trading of more kinds of related assets. Absolute accuracy levels, however, are not the key issue. The key policy question about any institution is how it performs relative to alternative institutions dealing with the same situation. A few studies have presented field data on this question, directly comparing real world speculative marketswithother realworldinstitutionsfor aggregatinginformation. Forexample, racetrack market odds improve on the prediction of racetrack experts (Figlewski, 1979). Florida orange juice commodity futures improve on government weather forecasts (Roll, 1984), Oscar markets beat columnist forecasts (Pennock, Giles, & Nielsen, 2001), and gas demand markets beat gas demand experts (Spencer, 2004). Betting markets beat major national opinion polls 451outof596timesinpredictingU.S.presidentialelectionresults(Berg&Rietz, 2002). Finally, betting markets beat Hewlett Packard official forecasts 6 times out of 8 at predicting Hewlett Packard printer sales (Chen & Plott, 2002; Plott, 2000). Unfortunately, no studies have directly compared estimates from speculative markets to estimates from academic-style institutions We do know, however, that those who do best at betting on horse races are smart in ways they can not articulate, and in ways unrelated to I.Q. (Ceci & Liker, 1986). Academic-style institutions, in contrast, seem largely limited to 6
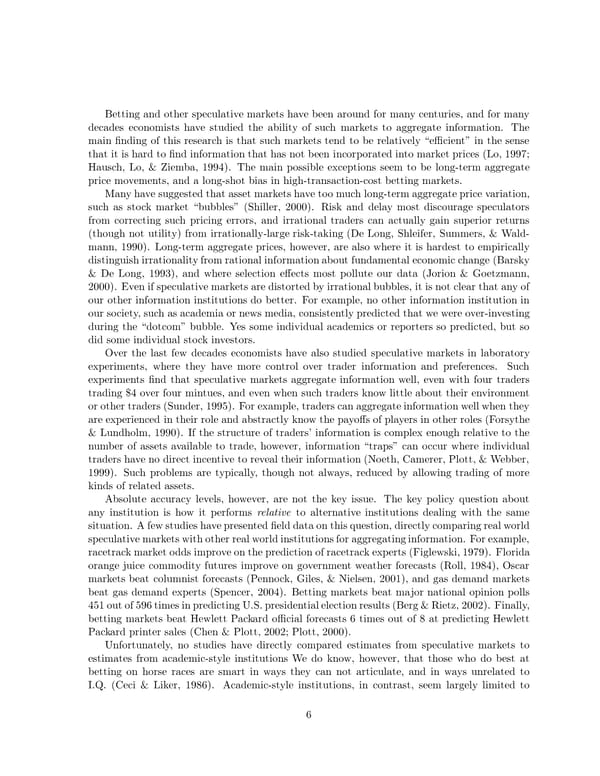